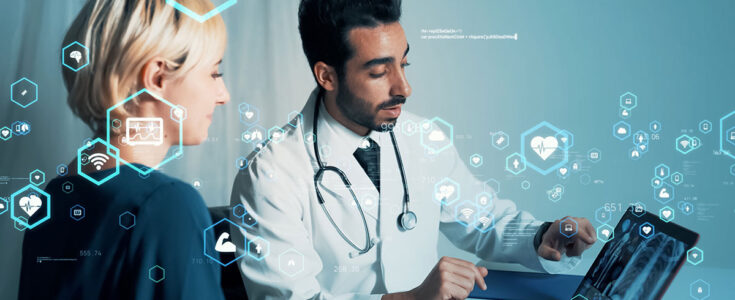
Generative AI has impacted our day-to-day life significantly. It excels at creating text responses based on Large Language Models. However, the accuracy of information provided by Generative AI continues to be questionable. Generative AI responses depend significantly on the LLMs they are trained on. The information on LLM may not be completely authenticated and updated, which forces organizations to be highly cautious while using a Generative AI model, specifically in industries such as Healthcare and Life Sciences.
Retrieval Augmented Reality (RAG) is a solution to this industry-agnostic challenge. RAG provides authenticated and updated information based on context provided to the model for generating answers, without changing the underlying LLM model. So, an organization can create its own RAG Architecture, which can help an existing LLM model to get more relevant, updated, and contextual information, providing more accurate results.
Also Read: Harnessing Generative AI in Cloud Migration: A Game-Changer for the 7 Rs Strategy
The potential of Generative AI, particularly RAG Architecture, to revolutionize healthcare and life sciences is undeniable. From expediting diagnoses and optimizing drug discovery to empowering patients and enhancing information retrieval, AI has the potential to transform healthcare by optimizing both administrative functions and medical care provisions. It will have financial and non-financial benefits for the global health system, such as improved care quality, enhanced patient experience, and greater clinician satisfaction.
Generative AI encompasses a broad range of techniques, including deep learning models and transformers, that can learn from existing data to generate entirely new content, such as text, images, and even code. This opens doors to a plethora of applications.
Retrieval-Augmented Generation (RAG) is the process of optimizing the output of a large language model, so it references an authoritative knowledge base outside of its training data sources before generating a response. At the core of RAG Architecture lie sophisticated architectures that facilitate seamless integration of retrieval and generation processes. These models leverage pre-trained language representations and employ mechanisms for information retrieval, comprehension, and synthesis, allowing them to comprehend queries, retrieve pertinent information, and generate coherent and contextually relevant responses.
Also Read: Generative AI in BFSI: A Real-World Success Story
Retriever: This component is responsible for efficiently retrieving relevant information from large databases or knowledge graphs based on the input query. It employs techniques such as semantic search or passage retrieval to identify and extract pertinent information.
Reader: Once the relevant information is retrieved, the reader component processes and comprehends the retrieved content, extracting key insights and understanding its context. This enables the model to generate contextually relevant responses aligned with the input query.
Also Read: From Science Fiction to Reality: The Rise of AI Digital Twins
Generator: Finally, the generator component synthesizes the retrieved information with the input query to generate coherent and contextually appropriate outputs. This component leverages techniques such as natural language generation (NLG) to produce human-readable text or other forms of content.
Overall, the integration of these components within the architecture of RAG Architecture empowers them to efficiently retrieve, comprehend, and generate information, making them invaluable tools for a wide range of applications in healthcare and life sciences.
The potential applications of RAG Architecture within the healthcare and life sciences are vast and transformative. Let’s explore some key use cases:
Also Read: Top 3 Use Cases of AI From a Healthcare Technology Leader
AI-enabled solutions could support clinicians in making precise diagnoses using inputs from in-vitro diagnostics, imaging, electronic health records (EHRs), patient conversations, biometrics, images, sensors, wearables, and genomics. RAG Architecture, with their ability to retrieve and integrate relevant information, can augment this process by providing healthcare professionals with comprehensive insights and supporting evidence for accurate diagnoses.
While concerns about data security remain valid, investments in secure data environments (SDEs) and robust data protection measures can alleviate these anxieties.
Also Read: Transformative Power of AI and Intelligent Automation in Pharmacovigilance
Moving forward, it is essential to create collaboration among researchers, medical professionals, politicians, and AI developers. Working together, we can achieve the full potential of Generative AI and RAG Architecture to transform healthcare, enhance patient outcomes, and, ultimately, build a healthier future for everyone.
Also Read: Fine-Tuning AI as a Catalyst for Medical Innovation