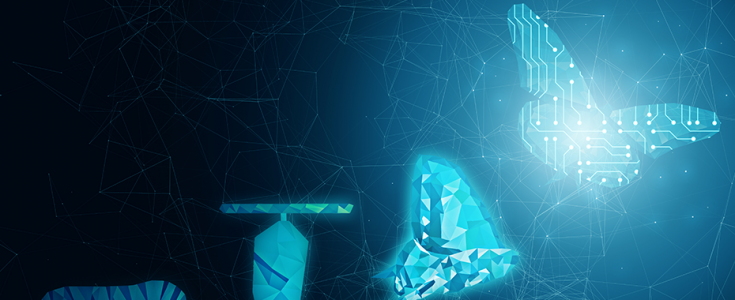
Widespread digital transformation has always been a matter of when and not if, but there is no doubt that it is now happening everywhere we look. And while required awareness has evolved at a relatively glacial pace, the increased use of data and analytics as a competitive differentiator is helping to drive business success.
In the globally connected village that the majority of us now live in, there are almost endless ways that organizations can wield data analytics to improve their offerings and customer engagement.
That all important USP might come in the form of a key customer-facing product feature, like information updates in real time or intuitive suggestions that enhance the end-user experience. Alternatively, analytics maybe driving operational improvements, like providing timely intel on stock, visibility into the supply chain or predicting future demand. In fact, digital transformation is increasingly becoming synonymous with data and analytics transformation.
Becoming a truly data-driven business requires shifts in how enterprises organize themselves, and the value of data and the role of chief data officer (CDO) is becoming increasingly important.
Until relatively recently, many organizations would put data officers primarily in charge of storing and securing data, rather than leading digital initiatives. And while a data-driven culture would appear to be a given in a connected society, those positions were not always mandatory – for, example, Gartner’s 2021 CDO Survey (the sixth to date) said that less than 50 percent of large companies have someone with the title of CDO.
Taking this number into account the integration of advanced data analytics is made possible by a perfect storm of factors, including but not limited to increasing digitization, rapid adoption of cloud, development in the cloud, microservices and technical advances in AI or ML. Although it’s always gratifying to see the strategic importance of data and analytics increasingly recognized at management and boardroom level, there is a consensus among the data community that this requires a strong technical foundation in order to deliver those strategic, valuable insights.
A Robust Base for Data-Driven Insights
Data is power, but only if you can take advantage of it. The challenge faced by many businesses is that they have a whole lot of data, but their ability to put it to work for the benefit of the business is constrained by increasing complexity, limited skill sets, and ill-equipped infrastructure.
Additionally, the amount of data they are dealing with is not only vast and continually growing, but it also comes in all shapes and sizes, both structured and unstructured. To be usable, it needs to be tamed.
As businesses get better at organizing their own data, they are looking to maximize their insights by combining their internal data with external data sources. For instance, the implications to public health of linking real-time internal data on hospital admissions with live pollution, weather or traffic data has far-reaching consequences for how we live and work.
These insights are not only within reach of companies with a proactive attitude to data and analytics but also capable of providing the requisite ROI within a short timeframe. The key point to remember is that the information is already available, what matters is how business leaders both access and optimize it.
Data Transformation: Walk Before You Run
For enterprises to get transformational outcomes from their data, they must understand and organize that data into a usable state. Importantly, they most also learn who owns the data itself.
This process of understanding, organization and learning is more commonly known as data transformation – the act of converting data from one format to another. Typically, data will come into an organization’s system from many different locations and in different formats, hence it will need to be standardized in accordance with the requirements of the destination system.
As we noted in a recent blog post, data transformation is an important step in the extract, transform and load (ETL) process.
Although there are many good, timesaving ETL tools available, it’s a stage that can end up being complex and time-consuming. Taking the opportunity to get this part of the ETL journey right is critical and it’s not hyperbole to say that your resulting data insights will be well worth it.
The basic process of transformation goes something like this:
The first step in the data transformation process consists of identifying and understanding the data in its source format. This is usually accomplished with the help of a data profiling tool. This step helps to identify data sources, validate the quality of the data, decide what changes needs to be performed to the data in order to get it into the desired state/format.
Think of this as the planning phase in which the transformation process is sketched out and agreed based on how the data sources are connected and the treatment they require.
In order for the transformation process to take place, code must be generated to run the transformation job. This stage is increasingly automated with the help of data transformation tools, but there can be cases when manual input is required.
The data is converted to the desired format. Often carried out in batches, the process that has been planned and coded is now put into action.
Transformed data needs to be checked to ensure it has been correctly formatted.
Depending on the size and complexity of the transformation job, this process can be repeated multiple times.
In addition, it may be necessary to customize operations further. Common customizations include data filtering (selecting only certain columns to load), enriching (splitting a column into multiples, as in going from Full Name to First Name, Middle Name and Last Name) and deduplicating. Furthermore, an important aspect for many data projects is how they handle data from external sources. Joining data from different sources will require a tailored approach.
Pros and Cons of Data Transformation
As we noted above, data transformation is the process by which organizations unlock the value of their data.
By investing in the right tools or solutions, companies can manage their data more effectively and ensure their data is high quality. This strategy means that the information can be used and re-used for multiple different data analytics projects to give meaningful results.
Data transformation also helps organizations perform faster queries. Reducing lag-time does not just benefit a single use case, for instance, but can even help open up new applications for analytics within an enterprise.
Given the benefits of data transformation, it seems like a no-brainer to conduct this part of a data project carefully. Yet, we need to be realistic about the challenges of data transformation in a typical enterprise scenario. Data transformation tasks often need to be fitted in around the many other urgent imperatives within the IT department.
Data transformation work can also be slow because processing large quantities of data puts a burden on other systems. We try to mitigate the disruptive effects by planning carefully when to process the data and ensuring it is carried out in batches.
It can also be time-consuming, depending on the scale of the project and the state of the data. The complaint you’ll hear most often from data scientists and analysts working on unstructured data is to do with how long this bit can take. That is why it is important to have clear parameters and a defined goal from the start and avoid scope creep.
Finally, cost can be an inhibitor. Large, complex projects will likely require a team of data experts, and that type of expertise can be hard to find – a recent report by Deloitte cited data science and analysis skills as not only being in-demand but also difficult to source when everybody is looking for this kind of talent.
All of these defined challenges mean that the actual act of data transformation can be delayed.
For companies that are looking to start the process now, the options are either wait until they know what they want to achieve or stumble along in the dark. The alternative to the latter option is to integrate a ready-made solution such as Gathi’s IC4™ Information Management offering – a cloud-native advanced data analytics platform that helps business leaders and analysts make smarter, faster decisions based on the foundational principles of information management (Curate, Catalog, Context, Consume).
Digital Transformation Starts with Data Transformation
If you think your data could be working harder for your organization, then it can be argued that data transformation must be a priority for 2022. Without wishing to hammer home the point, gathering, storing, securing and analyzing data is always time-intensive for any organization.
On the flip side, laying the right foundation for analytics is a path to digital success. And as automation grows within enterprises and AI-enhanced work becomes the norm, the right data base (pun intended) is an initiative well worth the investment.
Simply put, data-driven organizations are more innovative and more efficient than their less data-savvy counterparts. That is one reason why, according to Gartner, 33 percent of businesses are investing heavily in data-reliant initiatives like advanced analytics and AI.
Putting advanced data analytics to work depends on solid ETL capabilities with a strong focus on the data transformation phase. That requires an element of trust in proven methodologies and extensive data and analytics experience that will accelerate your organization’s path to digital maturity.
Data is now a commodity that companies must exploit to gain not only actionable insights but also engagement opportunities across the full spectrum of business verticals and stated digital goals. And while data has always been a competitive differentiator, the global village has made access to the right information at the right time the key element in the digital landscape.
Apexon’s teams of data architects and digital engineers are well-versed in the challenges that come with the required digital transformation, using our years of experience to deliver solutions to the questions that you should be asking. Thanks to our company-wide focus on data and analytics, we cover all the business, financial and technical aspects of data transformation to help entities gain the insights they need to speed digital product releases and improve efficiency.
If you need help transforming your disparate data into actionable intelligence, contact us by filling out the form below.